
1999) and by following the recommendation of Kameda ( 2012), which is to “mobilize “scientific imagination” in the process of decision”-by incorporating extreme events in risk modelling if no observations but sound scientific bases are available (Note that the term “reasoned imagination” is used by Paté-Cornell 2012). Our goal is specifically to capture and quantify extreme (i.e., low-probability–high-consequences) events using inductive generalization (e.g., Bier et al. Time-variant exposure supposes the evolution of assets value with time, which may be due to socioeconomic factors or to previous losses. More generally, time-variant vulnerability may refer to different processes, such as structure ageing, not-repaired pre-damage due to past events or damage conditioned on the co-occurrence of several events. Time-variant vulnerability and exposure related to hazard clustering are also considered, although not the primary focus of this study. Real-world examples of hazard interactions include: earthquake clustering, storm clustering, tsunamis following earthquakes or landslides, landslides or fire following earthquakes, storm surges associated to hurricanes, technological accidents triggered by natural events (i.e., NaTech events). In the present study, we present a novel, generic, multi-risk framework based on the sequential Monte Carlo Method (MCM) to allow for a straightforward and flexible implementation of hazard interactions, which may occur in a complex system. These are (1) the absence of clear definitions and (2) the lack of information on the added value of multi-risk assessment”. ( 2014) noted that: “two areas are most problematic. Similarly, but based on feedback from civil protection stakeholders, Komendantova et al. ( 2012), “despite growing awareness of relations between hazards, still neither a uniform conceptual approach nor a generally used terminology is applied”. The first two points are generally solved in dedicated multi-risk projects at the national (e.g., HAZUS-MH, ), international (e.g., CAPRA, ) or private sector levels (e.g., Grossi and Kunreuther 2005 Schmidt et al.
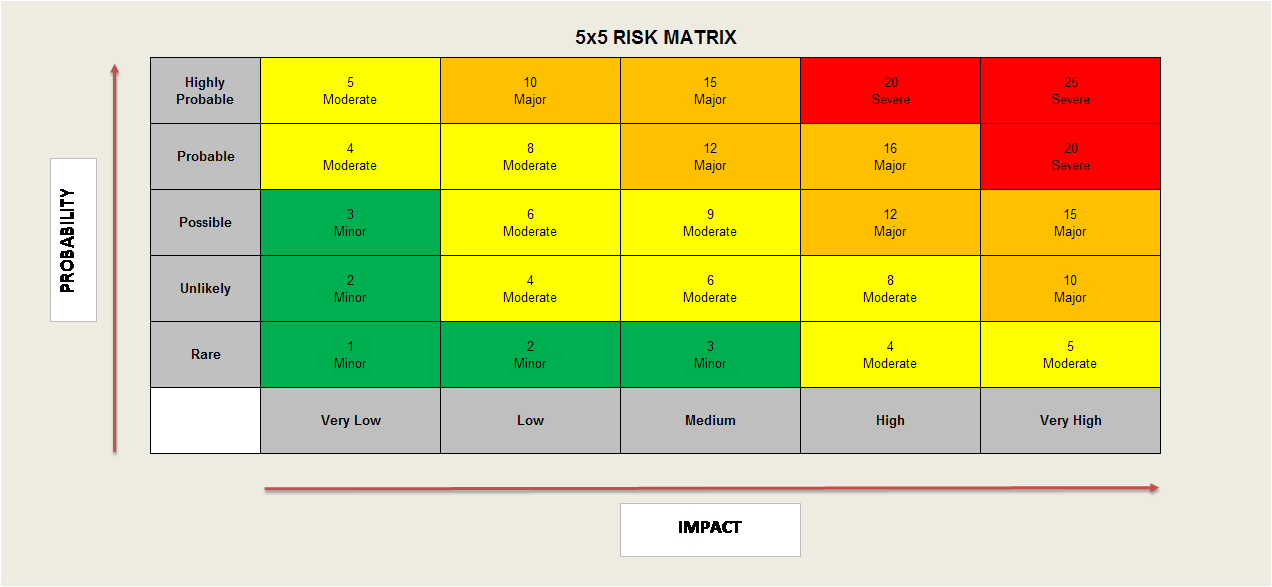
However, so far, only a limited number of scenario-based and/or site-specific multi-risk studies have been proposed due to the difficulty and novelty of the task.ĭevelopment of a comprehensive multi-risk framework is hampered by the following requirements: (1) large amount of input data (2) cross-disciplinary expertise and (3) innovative risk assessment methods. 2012) and of other dynamic aspects of risk, such as time-dependent vulnerability and exposure (e.g., Selva 2013) or network failures (e.g., Adachi and Ellingwood 2008). Innovative methods have been proposed in recent years to tackle the problem of hazard interactions (e.g., Marzocchi et al. Major catastrophes however remind us that multi-risk is not simply the sum of individual risks but that correlations between natural hazards, technological hazards and our complex socioeconomic networks lead to greater risks (e.g., 2005 hurricane Katrina, USA 2010 eruption of Eyjafjallajökull, Iceland 2011 Tohoku earthquake, Japan). Multi-risk assessment is still in its infancy and often only refers to the analysis of multiple single hazards in a same framework (Grünthal et al. We introduce the concept of risk migration matrix to evaluate how multi-risk participates to the emergence of extremes, and we show that risk migration (i.e., clustering of losses) and risk amplification (i.e., loss amplification at higher losses) are the two main causes for their occurrence. We find that extreme events can be captured by adding more knowledge on potential interaction processes using in a brick-by-brick approach.
#RISK PROBABILITY NUMBER IMPACT NUMBER SERIES#
Each simulated time series corresponds to one risk scenario, and the analysis of multiple time series allows for the probabilistic assessment of losses and for the recognition of more or less probable risk paths, including extremes or low-probability–high-consequences chains of events. We consider generic perils based on analogies with real ones, natural and man-made. We present a generic probabilistic framework based on the sequential Monte Carlo Method to implement coinciding events and triggered chains of events (using a variant of a Markov chain), as well as time-variant vulnerability and exposure. While much attention has been given to this aspect lately, most studies remain limited to a small number of site-specific multi-risk scenarios. Dynamic risk processes, which involve interactions at the hazard and risk levels, have yet to be clearly understood and properly integrated into probabilistic risk assessment.
